Artificial Intelligence and Machine Learning: Applying Advanced Tools for Public Health
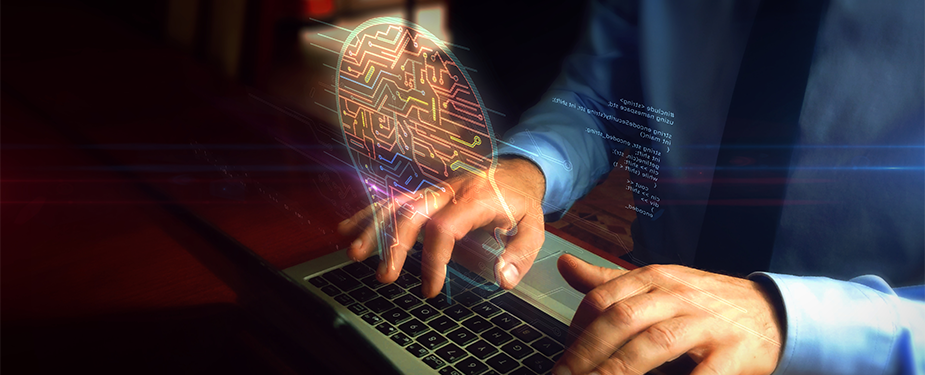
CDC’s Data Modernization Initiative supports artificial intelligence (AI), machine learning (ML) and other powerful solutions for large or complex data. These solutions can help us maximize insights from our data and systems and use those insights to drive public health action.
Machine learning (ML) allows a computer to analyze data to do a task without being explicitly programmed. The main kinds of machine learning are (1) to find patterns, like groupings of similar items and (2) to guess or predict an output based on a set of inputs.
Artificial intelligence (AI) applies technology to make computers (seem to) act rationally. In current practice, most AI is based on ML.
Why use AI/ML in public health?
AI/ML can help process massive amounts of data that are hard for humans to do at scale, across different modalities like images, audio, free text, genomic data, and others. It also helps us discover relationships in the data that are hard for traditional methods to find.
What has been done so far?
We are already seeing the benefits of using novel approaches to public health data. This work touches many different diseases and conditions and is helping public health become more responsive, accurate, and equitable.
For example, so far CDC has been able to:
- Improve speed and accuracy in surveillance by automatically detecting tuberculosis from chest X-rays
- Accelerate outbreak response to Legionnaires’ disease and prevent future disease by automatically detecting cooling towers from aerial imagery
- Enhance COVID-19 vaccine safety monitoring by using natural language processing (NLP) methods to analyze massive amounts of free text for potential safety signals
- Use more of the data we have:
- Identify opioid-related terms on death certificates, even if they’re misspelled
- Impute missing data from surveys, or fix sparsity in geographical sampling
- Use non-traditional data sources, including images, audio, social media, and data not specifically collected for public health analysis, such as electronic health records
- Be more mindful of potential disparities by evaluating fairness and mitigating bias in machine learning and other data-analytic methods
- Optimize case definitions for more accurate and efficient surveillance
- Discover patterns in clinical data and identify predictors for clinical outcomes
At CDC, the National Vital Statistics System has completed implementation of MedCoder, a new system that integrates natural language processing and machine learning for coding multiple causes of death. MedCoder can code nearly 90% of records automatically, compared to less than 75% for the previous system.
What's next?
CDC is exploring new applications of AI/ML for public health, including:
- Forecasting trends in opioid overdose mortality using heterogeneous data sources
- Syndromic surveillance using large language models and spatiotemporal point processes
- Using NLP methods on foodborne outbreak data to identify potential outbreak sources
- Detecting changes in inhabited areas from satellite imagery to streamline polio vaccine delivery in Nigeria
- Identifying personally identifiable information (PII) and protected health information (PHI) from unstructured text
CDC’s Center for Surveillance, Epidemiology, and Laboratory Services (CSELS) and National Center for Immunization and Respiratory Diseases (NCIRD) collaborated with UC Berkeley to develop a web application, TowerScout, to automatically detect cooling towers from satellite imagery. This tool is currently being used by the Legionnaires’ disease team and accelerates CDC’s ability to respond to outbreaks, potentially preventing additional illnesses and deaths.
Innovation and partnership
CDC has also worked closely with academic and technology partners to apply innovative approaches to common public health data challenges. For example, CDC and Georgia Tech Research Institute (GTRI) worked alongside state public health partners to
- Increase interoperability of mortality data and systems
- Integrate siloed systems and data streams for better analytical capabilities
- Connect disconnected data tools and systems for scale-up during response
- Improve our ability to capture and track data on exposures and health of vulnerable populations during emergencies
In addition, the CDC Data Hub actively continues to ensure that analytics, including ML/AI, are enabled in cloud-based data pipelines.
CDC has continued advancing the adoption of machine learning and artificial intelligence at the agency by directly funding projects involving AI and ML, as well as by sponsoring workforce training activities that will build the skills of staff in these areas. For example, CDC collaborates with the Council of State and Territorial Epidemiologists to offer the Data Science Team Training Program for health departments. Within CDC, the Data Science Upskilling@CDC fellowship program includes AI and ML training. In addition, other learning programs and networking activities strengthen CDC staff competencies in these areas.