Key points
Describes an alternative method to capture-recapture to estimate missing cases in Ohio’s ALS Registry, 2016-2018. Authors propose spatial analysis as an alternative means for estimating missing cases in disease registries.
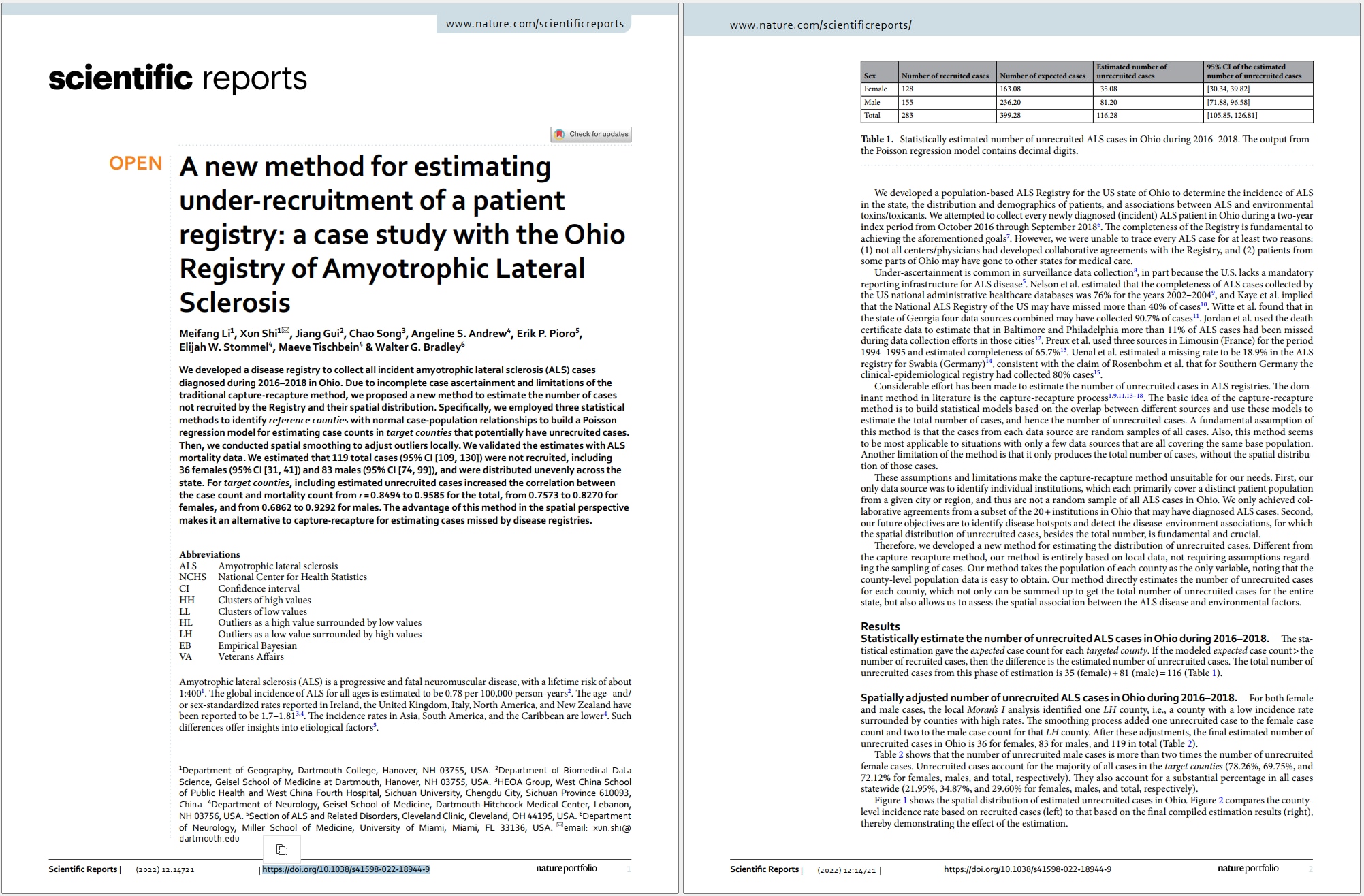
Affiliates
Meifang Li [1], Xun Shi [1], Jiang Gui [2], Chao Song [3], Angeline S. Andrew [4], Erik P. Pioro [4], Elijah W. Stommel [4], Maeve Tischbein [5] & Walter G. Bradley [6]
- Department of Geography, Dartmouth College, Hanover, NH, USA
- Department of Biomedical Data Science, Geisel School of Medicine at Dartmouth, Hanover, NH, USA
- HEOA Group, West China School of Public Health and West China Fourth Hospital, Sichuan University, Chengdu City, Sichuan Province, China
- Department of Neurology, Geisel School of Medicine, Dartmouth-Hitchcock Medical Center, Lebanon, NH, USA
- Section of ALS and Related Disorders, Cleveland Clinic, Cleveland, OH, USA
- Department of Neurology, Miller School of Medicine, University of Miami, Miami, FL, USA
Summary
This paper describes an alternative method to capture-recapture to estimate missing cases in Ohio’s ALS Registry. The team used statistical modeling and spatial adjustments to identify counties in Ohio between 2016-2018 with normal case-population relationships and from it built a methodology of identifying missing cases per county. Due to factors such as incomplete case ascertainment and the limitations inherent in capture-recapture, this paper proposes using spatial analysis as an alternative means for estimating missing cases in disease registries.